New Publication: Segmentation / Detection of Plant Roots in Minirhizotron and Rhizotron RGB Images

Exciting new research by Vienna Scientifics' Pavel Baykalov and Boris Rewald on root detection methodology in Minirhizotron, Rhizobox and Rhizotron / Root Window RGB root and rhizosphere images.
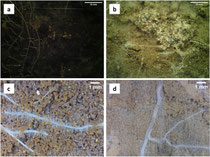
Manual analysis of mini-rhizotron images is time-consuming. While several methods exist for root segmentation in single-source datasets, recent advances in deep learning allow for automated feature extraction. This study compared six methods and proposes two improved deep learning models for automated, semantic root segmentation. The evaluation included performance on maize and various other species in minirhizotron and rhizotron /root window datasets of several experiments (Fig. 1), demonstrating the generalisation potential of the models on an unseen dataset. The following methodologies were compared:
- Frangi Vesselness
- Adaptive Threshold
- Support Vector Machine (SVM)
- SegRoot
- UNetGNRes
- U-Net
The focus of the research was on accuracy, false positives and transferability of these different MR root image analysis approaches, and how to improve root detection with advanced U-Net models.
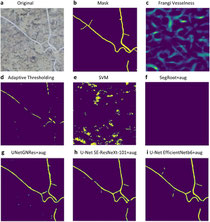
U-net models outperformed others methods, with increased encoder complexity improving root segmentation accuracy and generalisation potential (Fig. 2). The mixed MR dataset, composed of different image qualities, roots and soil conditions, challenged non-U-Net techniques, but data augmentation improved performance. Deep meta-architectures and feature extractors showed improved results and reduced false positives.
The research was carried out in and funded by the EU projects NextMR-IAA (ATTRACT) and FutureArctic ITN under grant agreements No. 777222 and 813114. We thank all colleagues for the great R&D collaboration within the projects and the input during the article preparation phase.
We are very interested in participating in research projects on root image segmentation - applying the developed methods to different root x soil sets and further improving the models - for the benefit of root science! Please contact us if you would like to discuss potential collaboration (including joint proposal writing) and how Vienna Scientific as an innovative SME can contribute its root segmentation / imaging expertise.
Reference & Download
Baykalov, P., Bussmann, B., Nair, R. et al. (2023). Semantic segmentation of plant roots from RGB (mini-) rhizotron images—generalisation potential and false positives of established methods and advanced deep-learning models. Plant Methods 19, 122. https://doi.org/10.1186/s13007-023-01101-2

Related VSI Products
Please see Minirhizotron corers for manual, tight installation of Minirhizotron observation tubes - reducing artefacts (voids, water droplets etc.) on MR images.
The Manual MS-190 Minirhizotron Camera System and Automatic AC-21 MR Camera provide unrivalled image quality - making root segmentation easier & more accurate.